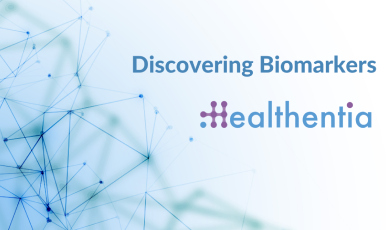
A biomarker is a naturally occurring characteristic by which a pathological or physiological process can be identified. A digital biomarker comprises of objective, quantifiable physiological and behavioral data, measured utilising digital portable, wearable, implantable or digestible devices, to be used to predict and manage health-related outcomes.
Innovation Sprint has built a composite contextual biomarker-based οn multiple aspects of Real-World Data (RWD), collected from people unobtrusively, while following-up their normal living routine. It is composite in the sense that it is not based on a single measurement, but rather on multiple diverse measurements (objective RWD) and peoples’ reports (subjective RWD). It is contextual in the sense that not only the person is measured, but also the person’s lifestyle context: social and environmental aspects complement the more traditional physiological and psychological ones.
Our RWD
At Innovation Sprint we are strong advocates of the empirical knowledge that lifestyle is a strong determinant of health. Hence our biomarker is based on RWD spanning four important aspects of a person’s lifestyle:
- Physiological RWD quantifies physical behaviour (active vs sedentary lifestyle as measured by steps walked, floors climbed, activity types, minutes in different intensity levels or heart rate zones, resting heart rate, sleep characteristics) and includes body info (height, weight, gender, race), nutrition (water, other liquids, food) and symptoms (body temperature, cough, diarrhea, headache, nausea, pain, etc.).
- Psychological RWD quantifies at a simple level mood, and in more complex situations mental state collected via elaborate, domain-specific questionnaires. Measurements can also play a role, either directly e.g. facial expression recognition or indirectly, e.g. weather where people are living).
- Social RWD quantifies social activity of people. This can be measured indirectly from the usage of the phone (diversity, duration, frequency of calls) and social media (diversity, number, frequency of interactions). More direct information can be reported using questionnaires on activities with friends, family or co-workers.
- Environmental RWD indicates the quality of life. Usually, reported by the users. Measurements of living or working environment quality are made with commercial devices (e.g. air quality meters).
AI for discovering our biomarker
Biomarker discovery at Innovation Sprint is done in three stages:
- Definition stage, where the domain experts select the clinically significant outcomes that need to be predicted by the biomarker(s).
- Manual RWD selection stage, where domain knowledge is applied to refine our generic RWD selection into those lifestyle aspects that are relevant to the disease/condition in question.
- Iterative design stage: Machine Learning/AI algorithms are used to train a proprietary classifier using the elected RWD to predict the selected clinically significant outcomes. The classifier is applied on new data yielding predictions and insights leading to digital therapeutics.
Validating our approach
We employed RWD collected over 7 years to train a biomarker that predicts significant weight changes. Such a biomarker is important for patients with several diseases (e.g. NAFLD), as well as for the general population interested in well-being. We achieved over 80% or correct prediction of the outcome, while we also analysed the different RWD aspects that led each individual to positive or negative outcomes, in order to offer personalized coaching services.
As we speak, we are utilising the same approach in other therapeutic areas, e.g. cervical cancer, to predict low toxicity events. Starting from 2021 we will validate this hypothesis in much larger cohorts, targeting –among others- COPD patients with Cardiovascular Disease comorbidities.
We will keep you update on our observations and findings!
Aristodemos Pnevmatikakis
R&D Director, Innovation Sprint