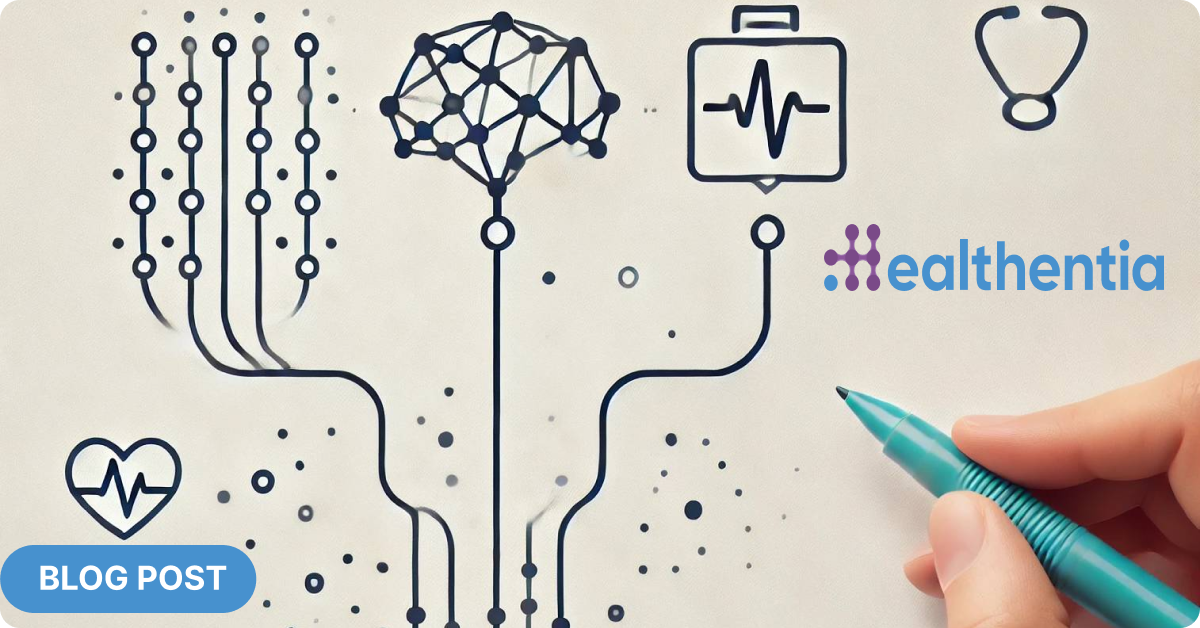
Our experiments indicate that model quality strongly depends on the data, both its volume and quality. Data volume is determined by the number of patients having already used Healthentia for a particular pathology. Data quality is determined by each patient’s adherence to the data collection plan. Both data volume and quality are seldom ideal. At least during the first studies Healthentia is used for a particular pathology, there are simply not enough patients to give us a satisfying volume of data. Moreover, patients are of varying degrees of capability to collaborate with Healthetnia’s mobile app. Omissions and errors can happen, and then reduce data quality.
Training with suboptimal data volume and quality leads to suboptimal models, suffering from overfitting and bias. The alternative is to reside to the knowledge of the experts, the healthcare professionals. Their knowledge is captured within rule-based expert systems. That yield the necessary analysis of the patients. At Innovation Sprint we have been experimenting with ways to combine the information the data offers and the expertise the healthcare professionals offer. The HumAIne project offers Healthentia the novel tools to achieve this combination.
HumAIne is an EU co-funded project under the topic HORIZON-CL4-2022-HUMAN-02-01 that started in October 2023. HumAIne facilitates advanced and reliable collaboration of experts and AI towards hybrid decision making and support in a variety of industries. It delivers the HumAIne Operating System, built on four technological pillars: Active Learning, Neuro-Symbolic Learning, Swarm Learning, and eXplainable AI. The HumAine OS enables AI solution creators to build advanced Human-AI collaboration systems that outperform standalone AI and individual experts’ efforts.
Innovation Sprint leads the project’s efforts on defining the vision and specifications for human-AI collaboration, focusing on user requirements extraction and use case scenarios definition. These efforts lead on the needs to be covered by the technology developed in HumAIne. We also lead the healthcare pilot, where the HumAIne technology will be leveraged to enhance the AI modules in the patient understanding and advice delivery systems of Healthentia. More specifically, we will be employing Neuro-Symbolic Learning to learn models not simply on data, but on the combined information of data and healthcare professionals’ expertise. We will be comparing the models learnt traditionally to those employing Neuro-Symbolic Learning, both in terms of prediction metrics but also in terms of their effectiveness in the overall behavioral change framework of Healthentia.